Credit Risk Modelling and Management
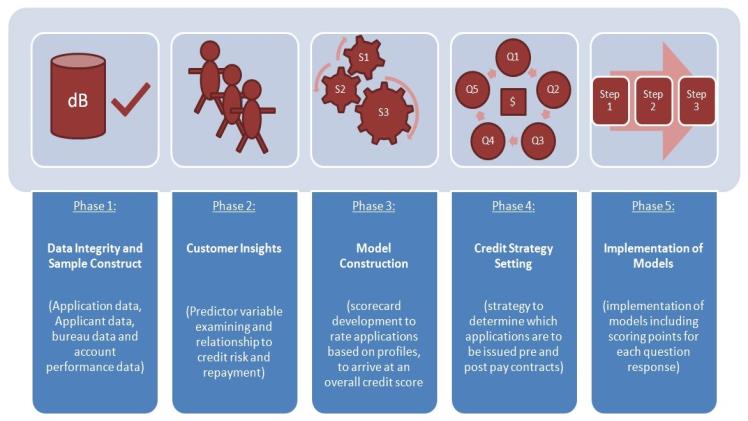
Credit Risk Modelling and Management refers to using data models to ascertain two important things: (1) the likelihood that a borrower will default on debt; and (2) its potential effect on lender finances should this happen. Financial institutions rely heavily on such models in making informed lending decisions.
Credit risk modelling is a highly complex endeavor and necessitates multiple advanced methodologies, including predictive models, optimization methods, mathematical models and mathematical simulations. The aim is to find an optimal model structure which maximizes return while minimising risk; lenders therefore must possess an in-depth knowledge of this aspect of lending operations management.
Probability of Default (POD) measures the likelihood that a borrower will fail to fulfill their loan repayment obligations as per agreed terms and conditions, and is the primary determinant of creditworthiness for individuals and corporations alike. POD depends on numerous factors including credit history, income and payment behavior of an individual – higher risk means higher interest rates or loan cancellation altogether, so when risk decreases lenders charge lower interest rates with better conditions. The more attractive an individual’s credit rating becomes and vice versa: lower risks mean better interest rate terms with better rates & terms offered to both parties involved – both individuals & corporations alike!
Alongside POD, factors that must be considered when assessing creditworthiness include collateral, cash flows and economic indicators. Credit risk models use all these aspects to estimate the probability that a borrower will default.
Click Here More Information: kpong krnl key and titfees
Institutions should regularly review and update their models in order to adapt to market changes or unexpected events, as well as ensure it works effectively across varying economic environments; otherwise it could fail to accurately predict credit risk.
Monitor and track the performance of a credit risk model regularly, in addition to conducting regular reviews. This will enable any discrepancies or biases within it to be identified quickly so they can be addressed before becoming risks to business operations.
To develop an accurate credit risk model, it requires high-quality data. To be useful, data must be accurate, consistent and current as well as well-structured and free from duplicates; to do this efficiently it may be beneficial to perform data quality tests before beginning modeling processes. Clearly define project goals with all stakeholders so as to ensure models are used effectively while adhering to regulations as well as measuring internal rating systems’ effectiveness and risk exposures over time.